
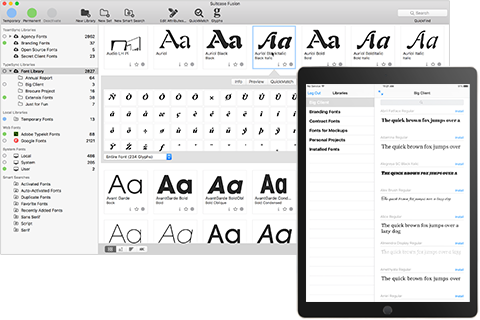

Chaudhuri, A Bayesian approach to visualization-oriented hyperspectral image fusion. Willsky, A multiscale approach to pixel-level image fusion.
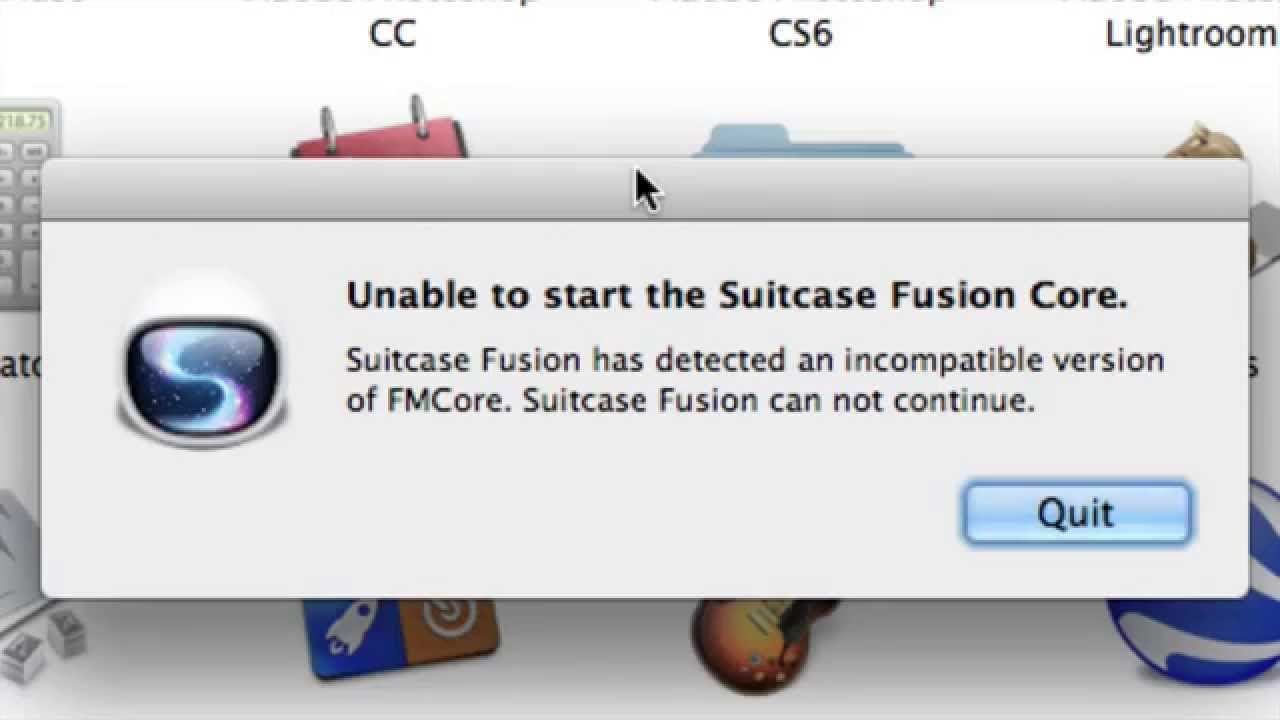
Giri, Introduction to Probability and Statistics, 2nd edn. Bogaert, Bayesian data fusion for adaptable image pansharpening. Escalante-Ramírez, The Hermite transform as an efficient model for local image analysis: an application to medical image fusion. Johnstone, Adapting to unknown smoothness via wavelet shrinkage. Chanda, Multi-focus image fusion using a morphology-based focus measure in a quad-tree structure. Chanda, A simple and efficient algorithm for multifocus image fusion using morphological wavelets. Kingsbury, Hidden Markov tree modeling of complex wavelet transforms, in Proceedings of the IEEE International Conference on Acoustics, Speech, and Signal Processing, vol. Abidi, A combinational approach to the fusion, de-noising and enhancement of dual-energy X-ray luggage images, in Proceedings of the IEEE Conference on Computer Vision and Pattern Recognition: Workshops, vol. Zhang, Multi-focus image fusion based on spatial frequency in discrete cosine transform domain. Kumar, A modified statistical approach for image fusion using wavelet transform. In addition, the proposed method yields excellent results in terms of visual quality even in the case of non-Gaussian noise as well as computational load. Results show that the performance of the proposed method is better than that of the other wavelet or principal component analysis-based methods in terms of various metrics such as the structural similarity, peak signal-to-noise ratio and cross-entropy, uses of which are common both in the areas of fusion and denoising. Experiments are carried out on a number of test-sets having an ensemble of multifocused source images with varying noise strengths to evaluate the performance of the proposed MAP-based fusion method as compared to the existing methods. A mathematically tractable multivariate a priori function is used in the MAP estimator to derive the closed-form expression of the fusion rule for the wavelet coefficients of noisy images. The central theme of the paper is to develop a novel generalized Bayesian framework based on maximum a posteriori (MAP) estimation technique to obtain the fused image from the noisy observations using a multiscale wavelet transform. This paper addresses the problem of simultaneous fusion and denoising of an ensemble of multifocused noisy source images using statistical approach.
